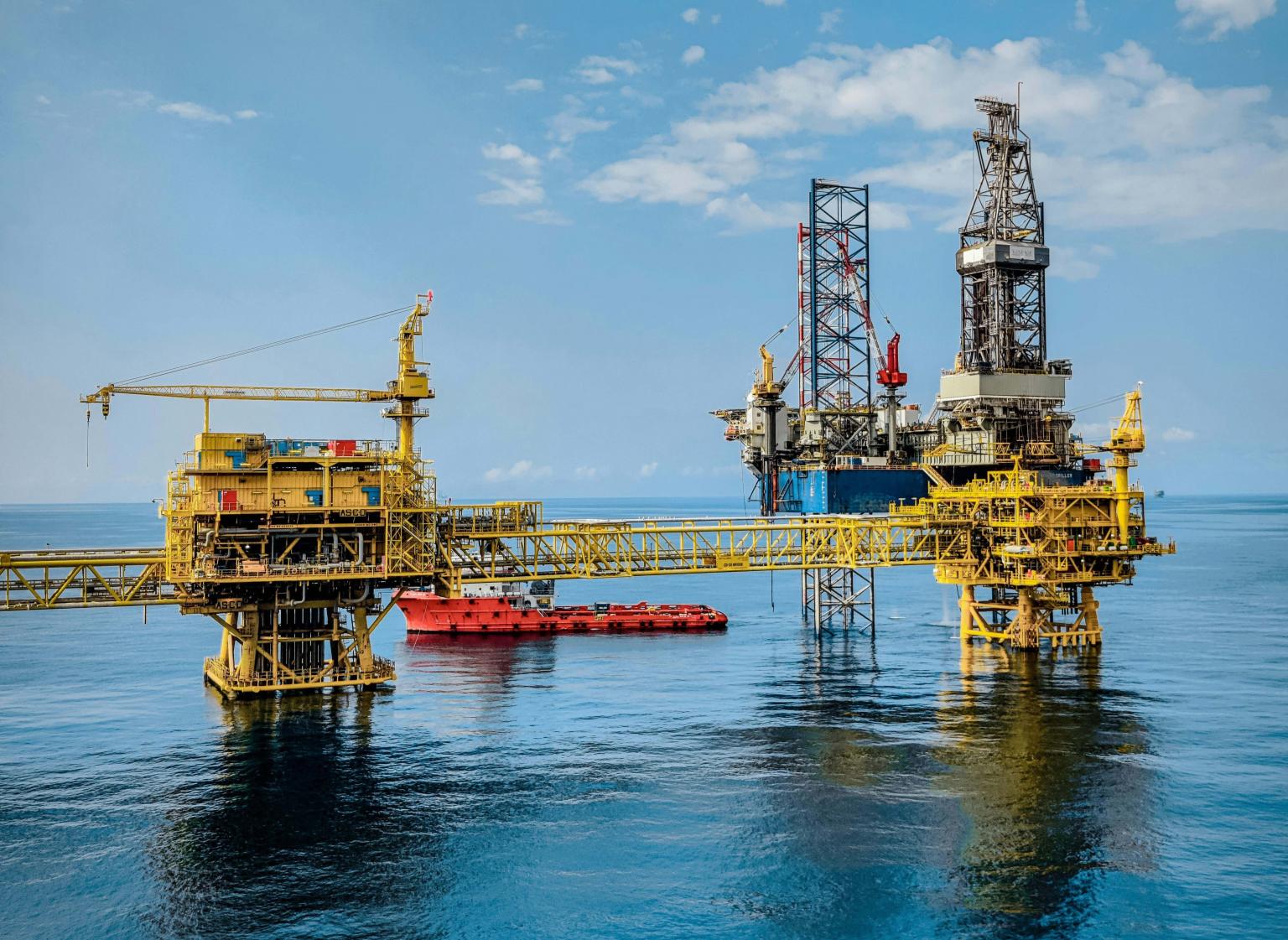
Artificial intelligence (AI), automation and machine learning are poised to revolutionise business operations in highly complex sectors, with upstream operations in the oil and gas industry serving as a prime example.
According to a recent report from Future Market Insights, the AI in Oil And Gas Market is estimated to be valued at US$ 3.5 billion in 2024 and is expected to reach US$13 billion by 2034, with a compound annual growth rate (CAGR) of 14.1% over that forecast period. Further underlining AI’s potential, the World Economic Forum indicates that large-scale adoption of AI in the oil and gas industry could account for 10–20% in cost savings by 2025.
This article will look at the key role AI could play in optimising critical business processes and unifying strategies around inventory, procurement, and maintenance.
Enhancing master data governance for optimal inventory management
Despite the immense financial investments in drilling, exploration and production, many organisations continue to grapple with inadequate planning. The root cause of this issue, typically, is poor master data governance.
Master data catalogues encompass information pertaining to wells, production facilities, the stores and parts inventories, and the assets and data that identify them. Typically, a major producer’s global catalogue might comprise 2-3 million items with an inventory value ranging from £2-4 billion. Nevertheless, despite its critical role in preserving system integrity and facilitating efficient business processes and analytics, the oil and gas sector frequently underestimates the significance of effective master data management.
This challenge is further exacerbated by the existence of isolated information scattered across various business units. Each entity or region has adopted its unique processes and nomenclature, potentially resulting in inconsistent data formats, duplication, information gaps, and other issues.
In tandem, well maintenance is typically administered at a regional level, with spares procurement on an ad hoc basis. Unfortunately, there is often a lack of comprehensive fleet-wide planning for critical parts held in inventory. For instance, a suitable specification bearing may be readily available locally, but due to cataloguing naming/format inconsistencies, an alternative part is sometimes transported from further afield.
Here, AI-enabled data analytics capabilities can rapidly help standardise component naming conventions, providing a global, holistic view of the inventory data. In a best practice example, they could autonomously pull data, enabling users to leverage insights at the right time for continuous improvement. While ensuring the health of assets, such an approach can also correlate historic operating temperature, pressure and maintenance data with production outages, revealing the most uptime-critical assets and helping to plan appropriate condition-based maintenance.
Enhanced visibility translates into heightened purchasing power
By using AI-analysis to unveil a comprehensive global perspective on critical parts requirements, purchasing power is increased. Procurement decisions can be standardised and consolidated. This enables producers to acquire enough components for all sites within a single order transaction, thereby maximising volume discounts, prior to distributing them to regional hubs. A 10 or 15% saving on a global inventory spend of £4-5m is certainly worth chasing. This approach also removes the internal cost of raising multiple regional purchase orders, which could be as much as £125-150 per order. With a clear understanding of their needs, organisations can even contemplate automating their annual global purchases on pre-agreed terms with select suppliers.
The Aberdeen Group estimates 50% of annual unscheduled asset downtime can be attributed to the lack of spare parts and stock outs. AI has a role to play in optimising and rightsizing the type and level of inventory held. Maintenance organisations usually establish a stocking strategy for critical spare parts when the asset is conceived. But this min/max safety buffer is an initial, often arbitrary projection – not yet based on real-world operational insight. This could result in safety stock levels that are 2-3 times higher than necessary throughout the asset’s lifetime, ultimately consuming working capital that cannot be recovered by the end of the asset’s life.
Through the implementation of AI, however, service and maintenance companies can analyse the real-world usage of components in similar operating scenarios. The outcome is a considerably more informed, and realistic stocking level recommendation, leading to substantial reductions in both inventory stockholding and costs. Case study data from Deloitte suggests inventory optimisation can reduce inventory carrying costs by 80%, raise material availability from 93 to 97+%, and generate savings of 20%.
How AI optimises drilling campaigns
Any offshore drilling campaign project needs an extensive supply of specialist piping together with warehoused drilling spares. These may represent stock worth trillions of pounds. However, as there is generally no holistic visibility or analysis of the existing drilling spares stockholding, the potential saving through re-deployment is typically not factored into the start of another new project.
AI provides a solution for examining drilling spares and warehousing inventory, aligning them with new project requirements. For instance, AI in conjunction with machine learning, could conduct cost analyses to establish if transferring existing inventory or procuring new items would be more cost-effective. Once more, AI promises better planning for supply chains to meet production targets efficiently.
It is also conceivable that we could use AI to orchestrate the purchasing and inventory management for various drilling campaigns as a capital project – for example, executing purchasing for a whole year’s activity in advance with a single, unified AI-driven system that seamlessly unites procurement project management with warehousing and inventory management.
AI role in carbon footprint reduction
Leveraging AI to help optimise production operations, encompassing maintenance and oil and gas extraction, will become increasingly important in minimising emissions stemming from well downtime or overproduction.
Given that oil fields typically yield both natural gas and oil, maintaining a delicate balance during extraction becomes paramount. In this scenario, AI can assist by forecasting and planning these highly complex extraction strategy decisions.
In the case of land-based wells, water and chemical tanks need to be emptied regularly to prevent the need for flaring, if gas is overproduced. By using the latest AI-enabled planning, scheduling and optimisation techniques, together with GPS data, water truck collections can be prioritised and routed to the most productive wells first, based on real-time needs.
AI’s holistic transformation
The integration of AI, automation, and machine learning is fundamentally reshaping the oil and gas industry, enabling companies to gain a holistic view of their operations. By removing data silos and harnessing AI’s power, organisations can optimise their buying power, refine stocking strategies, and achieve maximum uptime and productivity. Embracing AI is not just a technological leap; it’s a strategic move towards sustainable profitability and operational excellence in the ever-evolving landscape of the oil and gas sector.
Jeremiah Woodford is Vice President of Energy and Natural Resources at IFS.