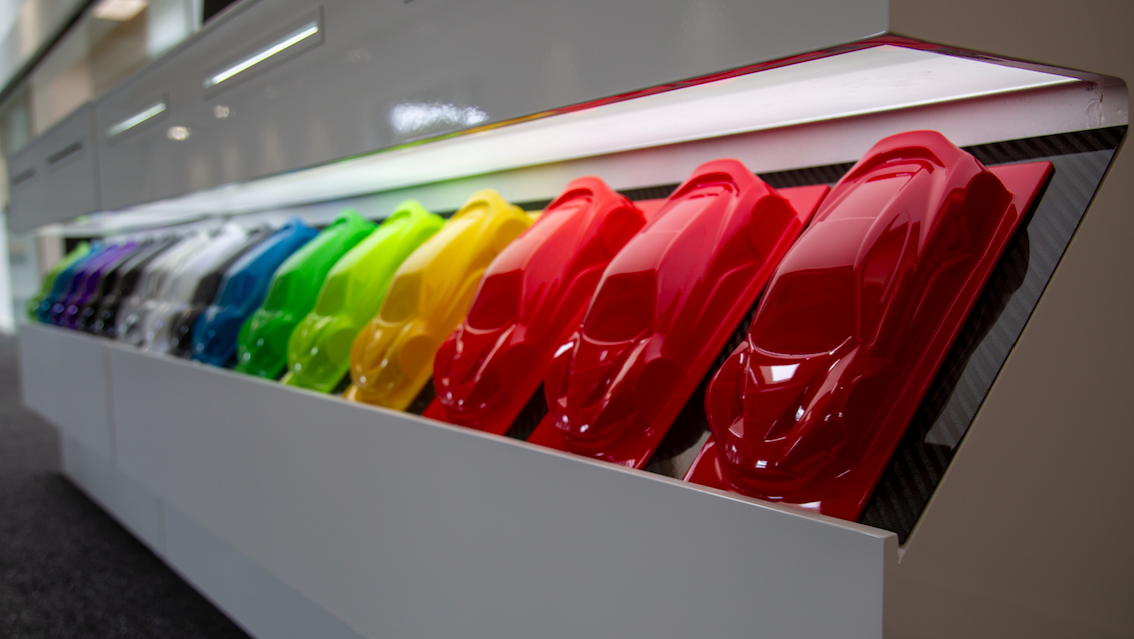
The transport industry has been making use of 3D printers for years – but while the machines and materials have changed over time, the techniques for developing those materials have not.
A research group at MIT, aided by BASF and Boston University, however, believes it has found a better method. Mike Foshey, a mechanical engineer and project manager in the Computational Design and Fabrication Group (CDFG) of the Computer Science and Artificial Intelligence Laboratory (CSAIL), explains, “Materials development is still very much a manual process: a chemist goes into a lab, mixes ingredients by hand, makes samples, tests them, and comes to a final formulation. But rather than having a chemist who is only able to create a couple of iterations over a span of days, our system can do hundreds of iterations over the same time span.”
The aim is to refine material parameters across numerous areas, such as strength in a certain direction or toughness. Foshey continues, “We think, for a number of applications, this would outperform the conventional method because you can rely more heavily on the optimisation algorithm to find the optimal solution. You wouldn’t need an expert chemist on hand to preselect the material formulations.”
At the heart of it all is machine learning. Rather than the laborious process above, the operator identifies a handful of ingredients, then keys in their chemical compositions into the algorithm. Then the final properties are specified and the algorithm adjusts the proportions appropriately to get a suitable end product. After the ingredients are mixed and tested, the results are fed back into the algorithm. There’s also a free software spin-off, AutoOED, which may be useful to researchers.
The team hopes this could even create new material options that human operators may overlook.
The best under test
To discover how well it was working, the researchers devised a test: perfecting an ink that sets under ultraviolet light. Toughness, compression modulus (stiffness), and strength were dialled in as final properties, as this particular combination features conflicting requirements that mean doing it manually would require getting one property at a time. Six materials were selected.
After a test run of 120 samples, 12 optimal options were created. Foshey says, “This has broad applications across materials science in general. For instance, if you wanted to design new types of batteries that were higher efficiency and lower cost, you could use a system like this to do it. Or if car manufacturers wanted to optimise paint that performed better and was environmentally friendly, this system could do that, too.”
Team member Keith A. Brown, assistant professor in the Department of Mechanical Engineering at Boston University adds, “The focus on novel material formulations is particularly encouraging as this is a factor that is often overlooked by researchers who are constrained by commercially available materials. The combination of data-driven methods and experimental science also allows the team to identify materials in an efficient manner. Because experimental efficiency is something with which all experimenters can identify, the methods here have a chance of motivating the community to adopt more data-driven practices.”